Land surface temperature predicts mortality due to chronic obstructive pulmonary disease: a study based on climate variables and impact machine learning
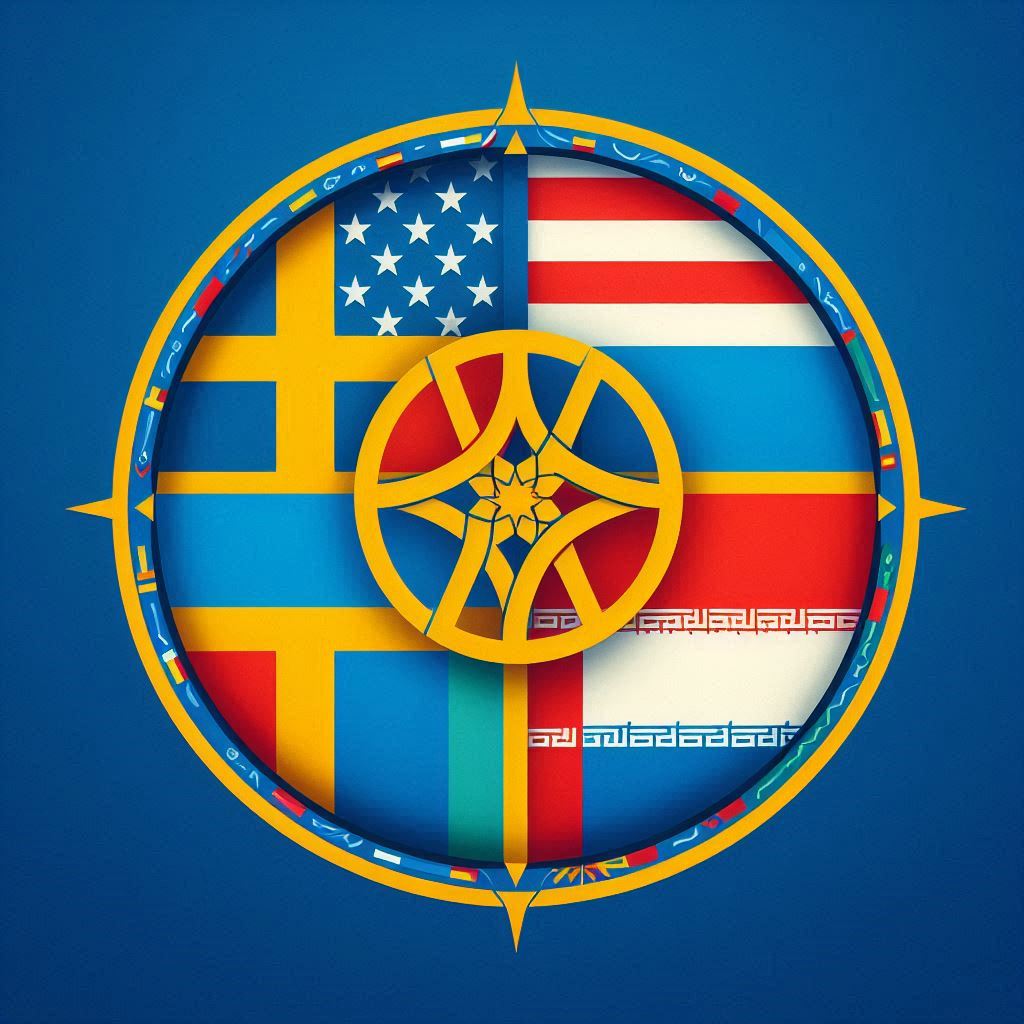
HTML: 0
All claims expressed in this article are solely those of the authors and do not necessarily represent those of their affiliated organizations, or those of the publisher, the editors and the reviewers. Any product that may be evaluated in this article or claim that may be made by its manufacturer is not guaranteed or endorsed by the publisher.
Authors
Introduction: Chronic Obstructive Pulmonary Disease (COPD) mortality rates and global warming have been in the focus of scientists and policymakers in the past decade. The long-term shifts in temperature and weather patterns, commonly referred to as climate change, is an important public health issue, especially with regard to COPD.
Method: Using the most recent county-level age-adjusted COPD mortality rates among adults older than 25 years, this study aimed to investigate the spatial trajectory of COPD in the United States between 2001 and 2020. Global Moran's I was used to investigate spatial relationships utilising data from Terra satellite for night-time land surface temperatures (LSTnt), which served as an indicator of warming within the same time period across the United States. The forest-based classification and regression model (FCR) was applied to predict mortality rates.
Results: It was found that COPD mortality over the 20-year period was spatially clustered in certain counties. Moran's I statistic (I=0.18) showed that the COPD mortality rates increased with LSTnt, with the strongest spatial association in the eastern and south-eastern counties. The FCR model was able to predict mortality rates based on LSTnt values in the study area with a R2 value of 0.68.
Conclusion: Policymakers in the United States could use the findings of this study to develop long-term spatial and health-related strategies to reduce the vulnerability to global warming of patients with acute respiratory symptoms.
How to Cite

This work is licensed under a Creative Commons Attribution-NonCommercial 4.0 International License.
PAGEPress has chosen to apply the Creative Commons Attribution NonCommercial 4.0 International License (CC BY-NC 4.0) to all manuscripts to be published.