Impact of socio-economic environment and its interaction on the initial spread of COVID-19 in mainland China
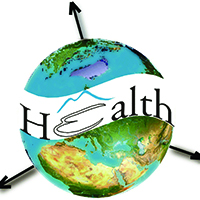
All claims expressed in this article are solely those of the authors and do not necessarily represent those of their affiliated organizations, or those of the publisher, the editors and the reviewers. Any product that may be evaluated in this article or claim that may be made by its manufacturer is not guaranteed or endorsed by the publisher.
Authors
Coronavirus disease 2019 (COVID-19) has strongly impacted society since it was first reported in mainland China in December 2020. Understanding its spread and consequence is crucial to pandemic control, yet difficult to achieve because we deal with a complex context of social environment and variable human behaviour. However, few efforts have been made to comprehensively analyse the socio-economic influences on viral spread and how it promotes the infection numbers in a region. Here we investigated the effect of socio-economic factors and found a strong linear relationship between the gross domestic product (GDP) and the cumulative number of confirmed COVID-19 cases with a high value of R2 (between 0.57 and 0.88). Structural equation models were constructed to further analyse the social-economic interaction mechanism of the spread of COVID-19. The results show that the total effect of GDP (0.87) on viral spread exceeds that of population influx (0.58) in the central cities of mainland China and that the spread mainly occurred through its interplay with other factors, such as socio-economic development. This evidence can be generalized as socio-economic factors can accelerate the spread of any infectious disease in a megacity environment. Thus, the world is in urgent need of a new plan to prepare for current and future pandemics.
How to Cite

This work is licensed under a Creative Commons Attribution-NonCommercial 4.0 International License.
PAGEPress has chosen to apply the Creative Commons Attribution NonCommercial 4.0 International License (CC BY-NC 4.0) to all manuscripts to be published.
Most read articles by the same author(s)
- Fazlay S. Faruque, Hui Li, Worth B. Williams, Lance A. Waller, Bruce T. Brackin, Lei Zhang, Kim A. Grimes, Richard W. Finley, GeoMedStat: an integrated spatial surveillance system to track air pollution and associated healthcare events , Geospatial Health: Vol. 8 No. 3 (2014)