A geographical information system model to define COVID-19 problem areas with an analysis in the socio-economic context at the regional scale in the North of Spain
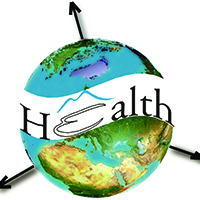
Accepted: 16 February 2022
HTML: 25
All claims expressed in this article are solely those of the authors and do not necessarily represent those of their affiliated organizations, or those of the publisher, the editors and the reviewers. Any product that may be evaluated in this article or claim that may be made by its manufacturer is not guaranteed or endorsed by the publisher.
Authors
The work presented concerns the spatial behaviour of coronavirus disease 2019 (COVID-19) at the regional scale and the socio-economic context of problem areas over the 2020-2021 period. We propose a replicable geographical information systems (GIS) methodology based on geocodification and analysis of COVID-19 microdata registered by health authorities of the Government of Cantabria, Spain from the beginning of the pandemic register (29th February 2020) to 2nd December 2021. The spatial behaviour of the virus was studied using ArcGIS Pro and a 1x1 km vector grid as the homogeneous reference layer. The GIS analysis of 45,392 geocoded cases revealed a clear process of spatial contraction of the virus after the spread in 2020 with 432 km2 of problem areas reduced to 126.72 km2 in 2021. The socio-economic framework showed complex relationships between COVID-19 cases and the explanatory variables related to household characteristics, socio-economic conditions and demographic structure. Local bivariate analysis showed fuzzier results in persistent hotspots in urban and peri-urban areas. Questions about €˜where, when and how' contribute to learning from experience as we must draw inspiration from, and explore connections to, those confronting the issues related to the current pandemic.
How to Cite

This work is licensed under a Creative Commons Attribution-NonCommercial 4.0 International License.
PAGEPress has chosen to apply the Creative Commons Attribution NonCommercial 4.0 International License (CC BY-NC 4.0) to all manuscripts to be published.
-
Eleonora Gioia, Alessandra Colocci, Cristina Casareale, Noemi Marchetti, Fausto MarincioniInternational Journal of Disaster Risk Reduction : 2022
-
Olga De Cos Guerra, Valentín Castillo Salcines, David Cantarero PrietoApplied Geography : 2024
-
Olga De Cos, Valentín Castillo, David CantareroJournal of Urban Health : 2023