Spatial correlates of COVID-19 first wave across continental Portugal
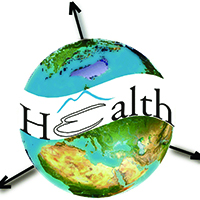
All claims expressed in this article are solely those of the authors and do not necessarily represent those of their affiliated organizations, or those of the publisher, the editors and the reviewers. Any product that may be evaluated in this article or claim that may be made by its manufacturer is not guaranteed or endorsed by the publisher.
Authors
The first case of COVID-19 in continental Portugal was documented on the 2nd of March 2020 and about seven months later more than 75 thousand infections had been reported. Although several factors correlate significantly with the spatial incidence of COVID-19 worldwide, the drivers of spatial incidence of this virus remain poorly known and need further exploration. In this study, we analyse the spatiotemporal patterns of COVID-19 incidence in the at the municipality level and test for significant relationships between these patterns and environmental, socioeconomic, demographic and human mobility factors to identify the mains drivers of COVID-19 incidence across time and space. We used a generalized liner mixed model, which accounts for zero inflated cases and spatial autocorrelation to identify significant relationships between the spatiotemporal incidence and the considered set of driving factors. Some of these relationships were particularly consistent across time, including the ‘percentage of employment in services’; ‘average time of commuting using individual transportation’; ‘percentage of employment in the agricultural sector’; and ‘average family size’. Comparing the preventive measures in Portugal (e.g., restrictions on mobility and crowd around) with the model results clearly show that COVID-19 incidence fluctuates as those measures are imposed or relieved. This shows that our model can be a useful tool to help decision-makers in defining prevention and/or mitigation policies.
How to Cite

This work is licensed under a Creative Commons Attribution-NonCommercial 4.0 International License.
PAGEPress has chosen to apply the Creative Commons Attribution NonCommercial 4.0 International License (CC BY-NC 4.0) to all manuscripts to be published.