Does mobility restriction significantly control infectious disease transmission? Accounting for non-stationarity in the impact of COVID-19 based on Bayesian spatially varying coefficient models
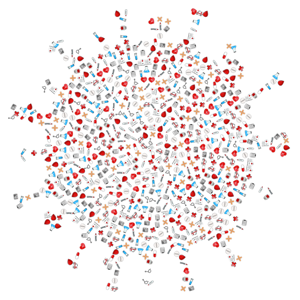
All claims expressed in this article are solely those of the authors and do not necessarily represent those of their affiliated organizations, or those of the publisher, the editors and the reviewers. Any product that may be evaluated in this article or claim that may be made by its manufacturer is not guaranteed or endorsed by the publisher.
Authors
COVID-19 is the most severe health crisis of the 21st century. COVID-19 presents a threat to almost all countries worldwide. The restriction of human mobility is one of the strategies used to control the transmission of COVID-19. However, it has yet to be determined how effective this restriction is in controlling the rise in COVID-19 cases, particularly in small areas. Using Facebook's mobility data, our study explores the impact of restricting human mobility on COVID-19 cases in several small districts in Jakarta, Indonesia. Our main contribution is showing how the restriction of human mobility data can give important information about how COVID-19 spreads in different small areas. We proposed modifying a global regression model into a local regression model by accounting for the spatial and temporal interdependence of COVID-19 transmission across space and time. We applied Bayesian hierarchical Poisson spatiotemporal models with spatially varying regression coefficients to account for non-stationarity in human mobility. We estimated the regression parameters using an Integrated Nested Laplace Approximation. We found that the local regression model with spatially varying regression coefficients outperforms the global regression model based on DIC, WAIC, MPL, and R2 criteria for model selection. In Jakarta's 44 districts, the impact of human mobility varies significantly. The impacts of human mobility on the log relative risk of COVID-19 range from –4.445 to 2.353. The prevention strategy involving the restriction of human mobility may be beneficial in some districts but ineffective in others. Therefore, a cost-effective strategy had to be adopted.
How to Cite

This work is licensed under a Creative Commons Attribution-NonCommercial 4.0 International License.
PAGEPress has chosen to apply the Creative Commons Attribution NonCommercial 4.0 International License (CC BY-NC 4.0) to all manuscripts to be published.