Sentiment analysis using a lexicon-based approach in Lisbon, Portugal
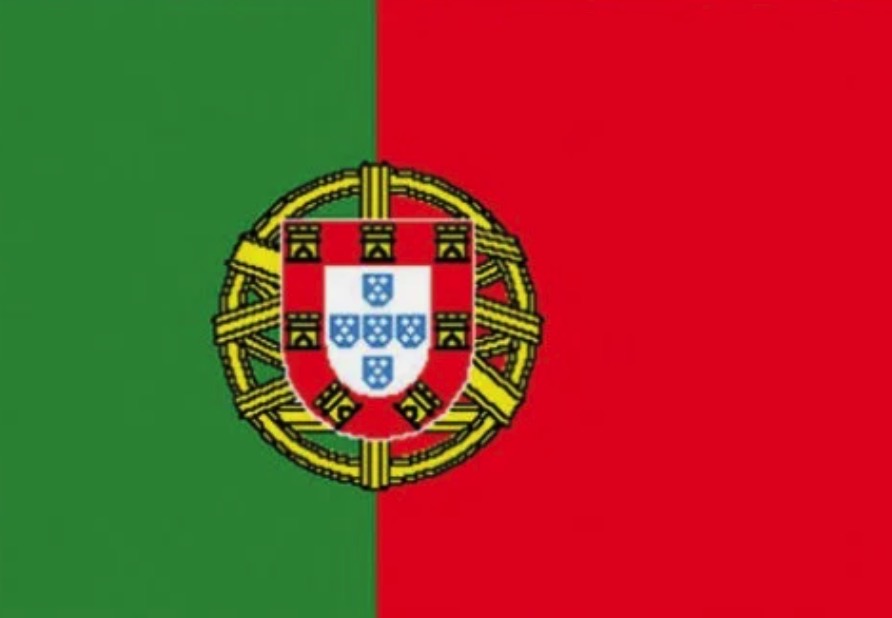
All claims expressed in this article are solely those of the authors and do not necessarily represent those of their affiliated organizations, or those of the publisher, the editors and the reviewers. Any product that may be evaluated in this article or claim that may be made by its manufacturer is not guaranteed or endorsed by the publisher.
Authors
Advances in digital sensors and Information flow have created an abundance of data generated by users under various emotional states in different situations. Although this opens up a new facet in spatial research, the large amount of data makes it difficult to analyze and obtain complete and comprehensive information leading to an increase in the demand for sentiment analysis. In this study, the Canadian National Research Council (NRC) of Sentiment and Emotion Lexicon (EmoLex) was used, based on data from the social network Twitter (now X), thus enabling the identification of the places in Lisbon where both positive and negative sentiment prevails. From the results obtained, the Portuguese are happy in spaces associated with leisure and consumption, such as museums, event venues, gardens, shopping centres, stores, and restaurants. The high score of words associated with negative sentiment have more bias, since the lexicon sometimes has difficulties to identify the context in which the word appears, ending up giving it a negative score (e.g., war, terminal).
How to Cite

This work is licensed under a Creative Commons Attribution-NonCommercial 4.0 International License.
PAGEPress has chosen to apply the Creative Commons Attribution NonCommercial 4.0 International License (CC BY-NC 4.0) to all manuscripts to be published.