Rapid identification of shallow inundation for mosquito disease mitigation using drone-derived multispectral imagery
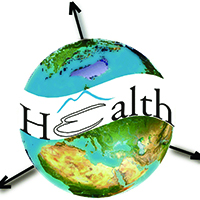
Accepted: 30 March 2020
Appendix: 159
HTML: 37
All claims expressed in this article are solely those of the authors and do not necessarily represent those of their affiliated organizations, or those of the publisher, the editors and the reviewers. Any product that may be evaluated in this article or claim that may be made by its manufacturer is not guaranteed or endorsed by the publisher.
Authors
Mosquito breeding habitat identification often relies on slow, labour-intensive and expensive ground surveys. With advances in remote sensing and autonomous flight technologies, we endeavoured to accelerate this detection by assessing the effectiveness of a drone multispectral imaging system to determine areas of shallow inundation in an intertidal saltmarsh in South Australia. Through laboratory experiments, we characterised Near-Infrared (NIR) reflectance responses to water depth and vegetation cover, and established a reflectance threshold for mapping water sufficiently deep for potential mosquito breeding. We then applied this threshold to field-acquired drone imagery and used simultaneous in-situ observations to assess its mapping accuracy. A NIR reflectance threshold of 0.2 combined with a vegetation mask derived from Normalised Difference Vegetation Index (NDVI) resulted in a mapping accuracy of 80.3% with a Cohen's Kappa of 0.5, with confusion between vegetation and shallow water depths (< 10 cm) appearing to be major causes of error. This high degree of mapping accuracy was achieved with affordable drone equipment, and commercially available sensors and Geographic Information Systems (GIS) software, demonstrating the efficiency of such an approach to identify shallow inundation likely to be suitable for mosquito breeding.
How to Cite
PAGEPress has chosen to apply the Creative Commons Attribution NonCommercial 4.0 International License (CC BY-NC 4.0) to all manuscripts to be published.
-
Frank Mechan, Zikmund Bartonicek, David Malone, Rosemary Susan LeesMalaria Journal : 2023
-
Gabriel Carrasco-Escobar, Marta Moreno, Kimberly Fornace, Manuela Herrera-Varela, Edgar Manrique, Jan E. ConnParasites & Vectors : 2022
-
Gheorghe-Gavrilă Hognogi, Ana-Maria Pop, Alexandra-Camelia Marian-Potra, Tania SomeșfăleanSustainability : 2021
-
Kenia Mayela Valdez-Delgado, David A. Moo-Llanes, Rogelio Danis-Lozano, Luis Alberto Cisneros-Vázquez, Adriana E. Flores-Suarez, Gustavo Ponce-García, Carlos E. Medina-De la Garza, Esteban E. Díaz-González, Ildefonso Fernández-SalasInsects : 2021
-
Andrew P. Colefax, Brendan P. Kelaher, Andrew J. Walsh, Cormac R. Purcell, Dan E. Pagendam, Daniele Cagnazzi, Paul A. ButcherBiological Conservation : 2021
-
K. T. Y. Mahima, Malith Weerasekara, Kasun De Zoysa, Chamath Keppitiyagama, Markus Flierl, Luca Mottola, Thiemo VoigtLecture Notes of the Institute for Computer Sciences, Social Informatics and Telecommunications Engineering : 2023
-
Andrée De Cock, Ruth Vandeputte, Stijn Bruneel, Laure De Cock, Xingzhen Liu, Rafael Bermúdez, Nina Vanhaeren, Bart De Wit, Daniel Ochoa, Philippe De Maeyer, Sidharta Gautama, Peter GoethalsDrones : 2023