Use of Twitter social media activity as a proxy for human mobility to predict the spatiotemporal spread of COVID-19 at global scale
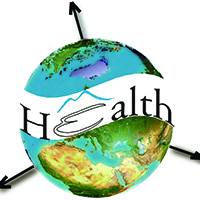
All claims expressed in this article are solely those of the authors and do not necessarily represent those of their affiliated organizations, or those of the publisher, the editors and the reviewers. Any product that may be evaluated in this article or claim that may be made by its manufacturer is not guaranteed or endorsed by the publisher.
Authors
As of February 27, 2020, 82,294 confirmed cases of coronavirus disease (COVID-19) have been reported since December 2019, including 2,804 deaths, with cases reported throughout China, as well as in 45 international locations outside of mainland China. We predict the spatiotemporal spread of reported COVID- 19 cases at the global level during the first few weeks of the current outbreak by analyzing openly available geolocated Twitter social media data. Human mobility patterns were estimated by analyzing geolocated 2013-2015 Twitter data from users who had: i) tweeted at least twice on consecutive days from Wuhan, China, between November 1, 2013, and January 28, 2014, and November 1, 2014, and January 28, 2015; and ii) left Wuhan following their second tweet during the time period under investigation. Publicly available COVID-19 case data were used to investigate the correlation among cases reported during the current outbreak, locations visited by the study cohort of Twitter users, and airports with scheduled flights from Wuhan. Infectious Disease Vulnerability Index (IDVI) data were obtained to identify the capacity of countries receiving travellers from Wuhan to respond to COVID-19. Our study cohort comprised 161 users. Of these users, 133 (82.6%) posted tweets from 157 Chinese cities (1,344 tweets) during the 30 days after leaving Wuhan following their second tweet, with a median of 2 (IQR= 1-3) locations visited and a mean distance of 601 km (IQR= 295.2-834.7 km) traveled. Of our user cohort, 60 (37.2%) traveled abroad to 119 locations in 28 countries. Of the 82 COVID-19 cases reported outside China as of January 30, 2020, 54 cases had known geolocation coordinates and 74.1% (40 cases) were reported less than 15 km (median = 7.4 km, IQR= 2.9-285.5 km) from a location visited by at least one of our study cohort's users. Countries visited by the cohort's users and which have cases reported by January 30, 2020, had a median IDVI equal to 0.74. We show that social media data can be used to predict the spatiotemporal spread of infectious diseases such as COVID-19. Based on our analyses, we anticipate cases to be reported in Saudi Arabia and Indonesia; additionally, countries with a moderate to low IDVI (i.e. ‰¤0.7) such as Indonesia, Pakistan, and Turkey should be on high alert and develop COVID- 19 response plans as soon as permitting.
How to Cite
PAGEPress has chosen to apply the Creative Commons Attribution NonCommercial 4.0 International License (CC BY-NC 4.0) to all manuscripts to be published.