Spatio-temporal modelling of tick life-stage count data with spatially varying coefficients
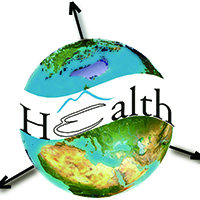
All claims expressed in this article are solely those of the authors and do not necessarily represent those of their affiliated organizations, or those of the publisher, the editors and the reviewers. Any product that may be evaluated in this article or claim that may be made by its manufacturer is not guaranteed or endorsed by the publisher.
Authors
There is a vast amount of geo-referenced data in many fields of study including ecological studies. Geo-referencing is usually by point referencing; that is, latitudes and longitudes or by areal referencing, which includes districts, counties, states, provinces and other administrative units. The availability of large geo-referenced datasets for modelling has necessitated the development and application of spatial statistical methods. However, spatial varying coefficients models exploring the abundance of tick counts remain limited. In this study we used data that was collected and prepared by researchers in the Department of Biological Sciences from the Old Dominion University, Virginia, USA. We modelled tick life-stage counts and abundance variability from 12 sampling locations, with 5 different habitats (numbered 1-5), three habitat types; namely: woods, edges and grass; collected monthly from May 2009 through December 2018. Spatio-temporal Poisson and spatio-temporal negative binomial (NB) count data models were fitted to the data and compared using the deviance information criteria (DIC). The NB model outperformed the Poisson models with all its DIC values being smaller than those of the Poisson model. Results showed that the covariates varied spatially across counties. There was a decreasing time (in years) effect over the study period. However, even though the time effect was decreasing over the study period, space-time interaction effects were seen to be increasing over time in York County.
How to Cite
PAGEPress has chosen to apply the Creative Commons Attribution NonCommercial 4.0 International License (CC BY-NC 4.0) to all manuscripts to be published.