Simulation of the spatial distribution of urban populations based on first-aid call data
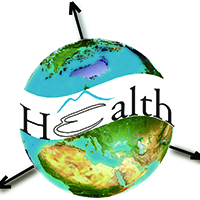
All claims expressed in this article are solely those of the authors and do not necessarily represent those of their affiliated organizations, or those of the publisher, the editors and the reviewers. Any product that may be evaluated in this article or claim that may be made by its manufacturer is not guaranteed or endorsed by the publisher.
Authors
We examined the feasibility of estimating the spatial distribution of urban populations based on first-aid calls based on one high-density place, the Shanghai urban area and one low-density place, the Nanhai District of Foshan City in Guangdong Province. We aggregated the population and the total number of first-aid calls on digital maps divided by grids based on a Geographic Information System (GIS). Geographically weighted regression was applied to test the correlation between the population distribution simulated by first-aid call data and the actual residency. The impact of different population densities, different grid cell sizes and different types of first-aid calls on simulation correlation were tested. We found that the use of first-aid call data could explain 60-95% of the actual population distribution in Shanghai using a grid with 1000*1000 m cell size, while the Nanhai experience was that first-aid calls could only explain 4-76% of the actual population distribution using a grid with 2000*2000 m cell size. Thus, the higher the population density, the better the simulation effect. For a high-population density area, the overall accuracy of simulation can reach as high as 0.878 at the 1-km2 resolution. However, there are different kinds of first-aid calls and for the best estimation of the population distribution in densely populated areas, we suggest using first-aid calls from people requiring acute medical care rather than all first-aid call data.
How to Cite

This work is licensed under a Creative Commons Attribution-NonCommercial 4.0 International License.
PAGEPress has chosen to apply the Creative Commons Attribution NonCommercial 4.0 International License (CC BY-NC 4.0) to all manuscripts to be published.